Model Optimization and Nonlinear Models#
CSC/DSC 340 Week 5 Slides
Author: Dr. Julie Butler
Date Created: August 19, 2023
Last Modified: September 18, 2023
Plan for the Week#
Monday
Week 5 Pre-Class Homework and Week 4 In-Class Assignment Due
Lecture: Model Optimization and Nonlinear Models
Office Hours: 1pm - 3pm
Tuesday
Office Hours: 4pm - 6pm
Wednesday
Finish Lecture: Model Optimization and Nonlinear Models
Start Week 5 In-Class Assignment
Week 4 Post-Class Homework Due
Thursday
Office Hours: 12:30 - 2pm
Friday
In-Class Assignment Week 5
Problem Analysis Due Before Class (~1 page)
Part 1: Hyperparameter Tuning#
Why do we need hyperparameter tuning?#
The values of the hyperparameters change the output of the model
Bad hyperparameters lead to bad results
##############################
## IMPORTS ##
##############################
import numpy as np
import matplotlib.pyplot as plt
from sklearn.linear_model import Lasso, Ridge
from sklearn.preprocessing import StandardScaler
from sklearn.model_selection import train_test_split
from sklearn.metrics import mean_squared_error as MSE
from sklearn.datasets import fetch_california_housing
New data set: California Housing
Goal: Predict the price a house will sale for (house price/100k) given information about the house
In-Class Week 5
# Print the features
fetch_california_housing().feature_names
['MedInc',
'HouseAge',
'AveRooms',
'AveBedrms',
'Population',
'AveOccup',
'Latitude',
'Longitude']
##############################
## IMPORT DATA ##
##############################
X, y = fetch_california_housing(return_X_y = True)
##############################
## SCALE DATA ##
##############################
scaler = StandardScaler()
scaler.fit(X)
Z = scaler.transform(X)
##############################
## TRAIN-TEST SPLIT ##
##############################
X_train, X_test, y_train, y_test = train_test_split(Z,y,test_size=0.2)
##############################
## GOOD ALPHA ##
##############################
ridge = Ridge(alpha=1e-15)
ridge.fit(X_train, y_train)
y_pred = ridge.predict(X_test)
err = MSE(y_pred, y_test)
print("MSE:", err)
X_test_plot = scaler.inverse_transform(X_test)
plt.scatter(X_test_plot[:,1],y_test,label='True')
plt.scatter(X_test_plot[:,1],y_pred,label='Predicted')
plt.legend()
MSE: 0.5207853811806975
<matplotlib.legend.Legend at 0x1379a6610>
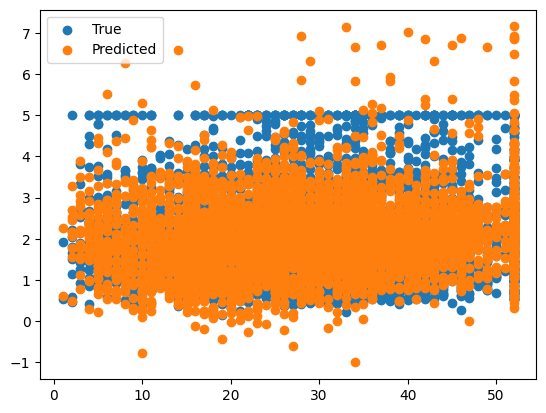
##############################
## BAD ALPHA ##
##############################
ridge = Ridge(alpha=1e15)
ridge.fit(X_train, y_train)
y_pred = ridge.predict(X_test)
err = MSE(y_pred, y_test)
print("MSE:", err)
X_test_plot = scaler.inverse_transform(X_test)
plt.scatter(X_test_plot[:,1],y_test,label='True')
plt.scatter(X_test_plot[:,1],y_pred,label='Predicted')
MSE: 1.3166823615106975
<matplotlib.collections.PathCollection at 0x137a58970>
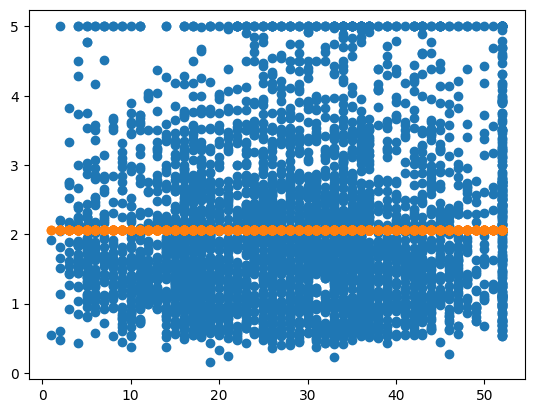
Methods for Hyperparameter Tuning#
Using Default Values#
Scikit-Learn sets the default value of \(\alpha\) for ridge regression to 1.0, which is a reasonable high level of regularization
# Make the data set smaller (20k+ points in total)
# More points = more data to generate patterns BUT more run time
X = X[:1000]
y = y[:1000]
X_train, X_test, y_train, y_test = train_test_split(X,y,test_size=0.2)
%%time
ridge = Ridge()
ridge.fit(X_train, y_train)
y_pred = ridge.predict(X_test)
CPU times: user 2.43 ms, sys: 5.11 ms, total: 7.54 ms
Wall time: 1.29 ms
err = MSE(y_pred, y_test)
print("MSE:", err)
X_test_plot = scaler.inverse_transform(X_test)
plt.scatter(X_test_plot[:,1],y_test,label='True')
plt.scatter(X_test_plot[:,1],y_pred,label='Predicted')
MSE: 0.3596000507155571
<matplotlib.collections.PathCollection at 0x137b13cd0>
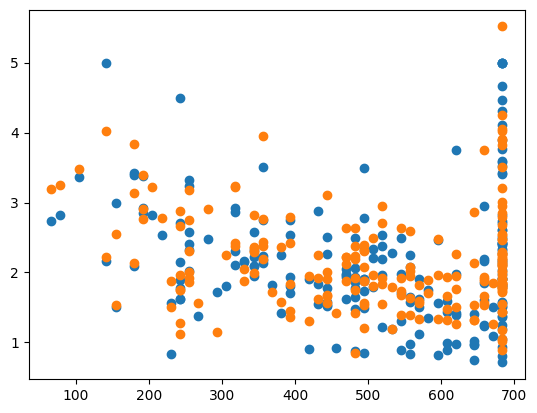
Pros#
Fast and no need to modify the algorithm
Cons#
Default value may not be the best value, but no test are done to check
For Loop Tuning#
Use a for loop to check many different values for the hyperparameters
Can use nested for loops if more than one hyperparamter
%%time
best_err = 1e4
best_alpha = None
for alpha in np.logspace(-15,4,1000):
ridge = Ridge(alpha=alpha)
ridge.fit(X_train, y_train)
y_pred = ridge.predict(X_test)
err = MSE(y_pred, y_test)
if err < best_err:
best_err = err
best_alpha = alpha
CPU times: user 630 ms, sys: 5.05 ms, total: 635 ms
Wall time: 297 ms
ridge = Ridge(alpha=best_alpha)
ridge.fit(X_train, y_train)
y_pred = ridge.predict(X_test)
err = MSE(y_pred, y_test)
print("MSE:", err)
print("CHOSEN ALPHA:", best_alpha)
X_test_plot = scaler.inverse_transform(X_test)
plt.scatter(X_test_plot[:,1],y_test,label='True')
plt.scatter(X_test_plot[:,1],y_pred,label='Predicted')
MSE: 0.3552471269777846
CHOSEN ALPHA: 6.022541201461928e-15
<matplotlib.collections.PathCollection at 0x1076fbb50>
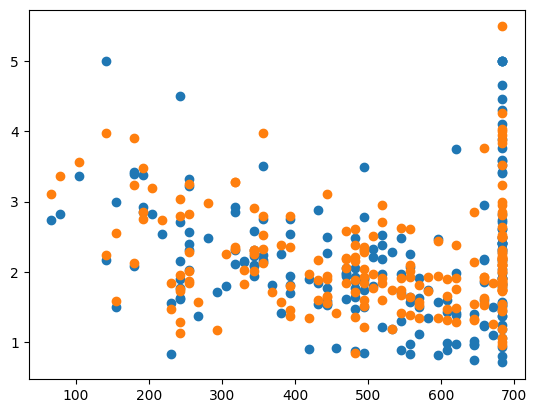
Pros#
Checks more than one value to find the best value
Simple concept
Short(ish) run times
Cons#
Long piece of code
Not checking all possible values of the hyperparameters
GridSearchCV (Scikit-Learn)#
Scikit-Learn has several hyperparameter tuning implementation
Grid search is a brute force algorithm which checks as many values as given
If more than one hyperparameter, it checks every single possible combination
“Same” test as for loops but gives much more information, but also takes longer
%%time
from sklearn.model_selection import GridSearchCV
parameters = {'alpha':np.logspace(-15,4,5000)}
ridge = Ridge()
grid_search = GridSearchCV(ridge, parameters,\
scoring='neg_mean_squared_error')
grid_search.fit(X_train, y_train)
print(grid_search.best_params_)
{'alpha': 0.20590302760544998}
CPU times: user 13.2 s, sys: 212 ms, total: 13.4 s
Wall time: 13.1 s
ridge = Ridge(alpha=grid_search.best_params_['alpha'])
ridge.fit(X_train, y_train)
y_pred = ridge.predict(X_test)
err = MSE(y_pred, y_test)
print("MSE:", err)
print('CHOSEN ALPHA:', grid_search.best_params_['alpha'])
X_test_plot = scaler.inverse_transform(X_test)
plt.scatter(X_test_plot[:,1],y_test,label='True')
plt.scatter(X_test_plot[:,1],y_pred,label='Predicted')
MSE: 0.3562628152996591
CHOSEN ALPHA: 0.20590302760544998
<matplotlib.collections.PathCollection at 0x137e80a90>
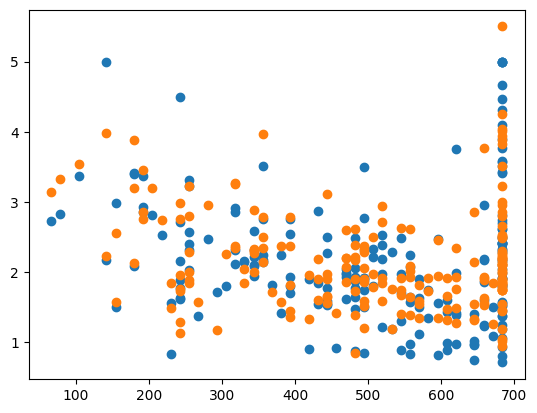
Pros#
Only takes a few lines to implement
Gives a lot of data once it is fit
Cons#
Long run times
Only searches the range of parameters given
RandomizedSearchCV (Scikit-Learn)#
Tries
n_iter
randomly chosen values for the hyperparmeters taken from a given distribution (uniform in this case)
%%time
from sklearn.model_selection import RandomizedSearchCV
from scipy.stats import uniform
distributions = {'alpha':uniform(loc=0, scale=4)}
ridge = Ridge()
random_search = RandomizedSearchCV(ridge, distributions,\
scoring='neg_mean_squared_error', n_iter=5000)
random_search.fit(X_train, y_train)
print(random_search.best_params_, random_search.best_score_)
{'alpha': 0.20597888239758078} -0.28856463563603973
CPU times: user 13.3 s, sys: 230 ms, total: 13.5 s
Wall time: 13.2 s
ridge = Ridge(alpha=random_search.best_params_['alpha'])
ridge.fit(X_train, y_train)
y_pred = ridge.predict(X_test)
err = MSE(y_pred, y_test)
print("MSE:", err)
print('CHOSEN ALPHA:', random_search.best_params_['alpha'])
X_test_plot = scaler.inverse_transform(X_test)
plt.scatter(X_test_plot[:,1],y_test,label='True')
plt.scatter(X_test_plot[:,1],y_pred,label='Predicted')
MSE: 0.35626318516764216
CHOSEN ALPHA: 0.20597888239758078
<matplotlib.collections.PathCollection at 0x164bb3940>
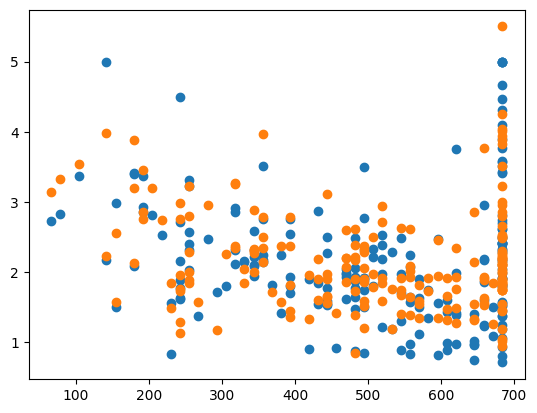
Pros#
Only takes a few lines to implement
Gives a lot of data once it is fit
Cons#
Long run times (depending on value of
n_iter
)Only searches a finite number of parameter combinations
Bayesian Ridge Regression#
Finds the “most likely” value for \(\alpha\) using Bayesian stastics
Leaves no uncertainity that the best value was just not sampled
%%time
from sklearn.linear_model import BayesianRidge
bayesian_ridge = BayesianRidge()
bayesian_ridge.fit(X_train, y_train)
y_pred = bayesian_ridge.predict(X_test)
print(bayesian_ridge.alpha_)
3.688440522864384
CPU times: user 5.58 ms, sys: 1.28 ms, total: 6.85 ms
Wall time: 1.68 ms
ridge = BayesianRidge()
ridge.fit(X_train, y_train)
y_pred = ridge.predict(X_test)
err = MSE(y_pred, y_test)
print("MSE:", err)
print('CHOSEN ALPHA:', ridge.alpha_)
X_test_plot = scaler.inverse_transform(X_test)
plt.scatter(X_test_plot[:,1],y_test,label='True')
plt.scatter(X_test_plot[:,1],y_pred,label='Predicted')
MSE: 0.3592175812997633
CHOSEN ALPHA: 3.688440522864384
<matplotlib.collections.PathCollection at 0x164d18610>
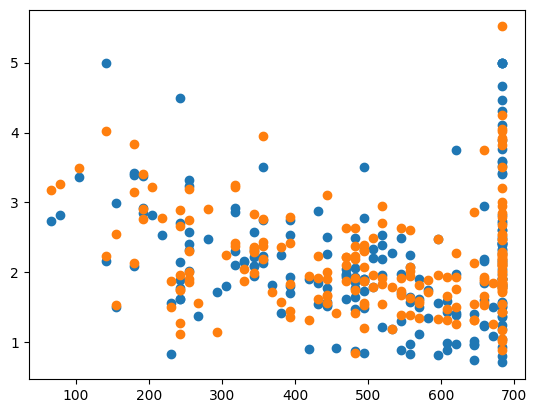
Pros#
Only takes a few lines to implement
Statical certainity that the given \(\alpha\) value is the best value
Short run times
Cons#
Only valid for Bayesian ridge regression (linear model)
Comparison of \(\alpha\), Accuracy, and Run Time#
Exact values depend on the train-test split and RandomizedSearch results; these values were taken from one run
labels = ['default', 'for loops', 'GridSearchCV', 'RandomizedSearchCV', 'Bayesian']
alphas = [1.0, 1e-15, 769.79, 3.99, 1.90]
mse = [0.23461613368850237, 0.2343672966024232, 0.23546465060931623, 0.23546192678932165, 0.23439940455100014]
run_times = [20.8/1000, 1.35, 57.7, 7*60+40, 1.18] #ms
plt.bar(np.arange(0,len(labels)), alphas)
plt.yscale('log')
plt.xticks(np.arange(0,len(labels)), labels, rotation=45)
plt.ylabel(r"Best $\alpha$ Value")
plt.xlabel("Hyperparameter Tuning Method")
Text(0.5, 0, 'Hyperparameter Tuning Method')
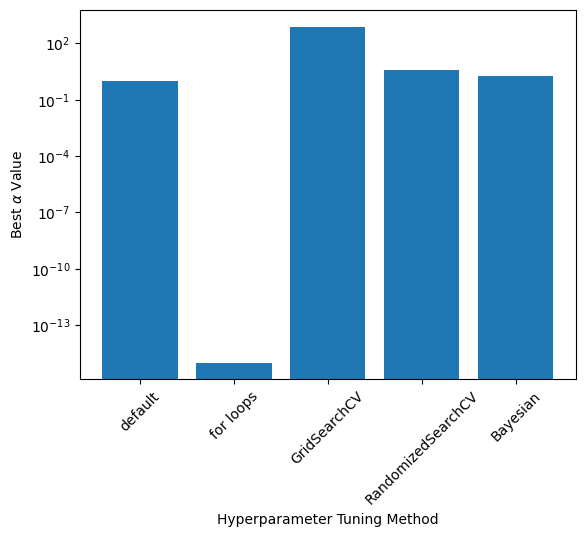
plt.bar(np.arange(0,len(labels)), mse)
plt.xticks(np.arange(0,len(labels)), labels, rotation=45)
plt.ylabel(r"MSE")
plt.xlabel("Hyperparameter Tuning Method")
Text(0.5, 0, 'Hyperparameter Tuning Method')
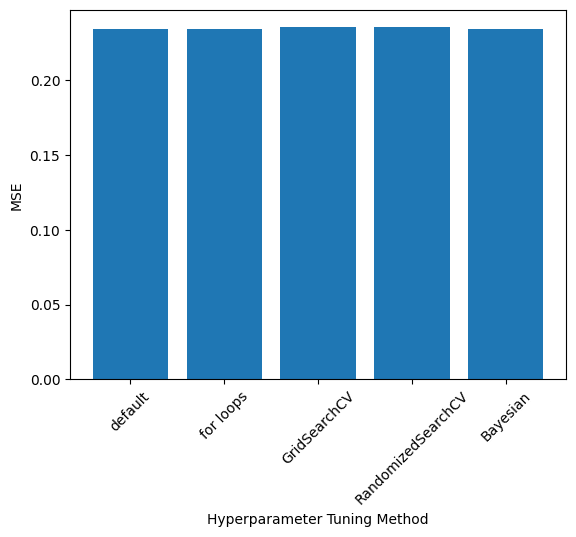
plt.bar(np.arange(0,len(labels)), run_times)
plt.xticks(np.arange(0,len(labels)), labels, rotation=45)
plt.ylabel(r"Run Time (sec.)")
plt.xlabel("Hyperparameter Tuning Method")
plt.yscale('log')
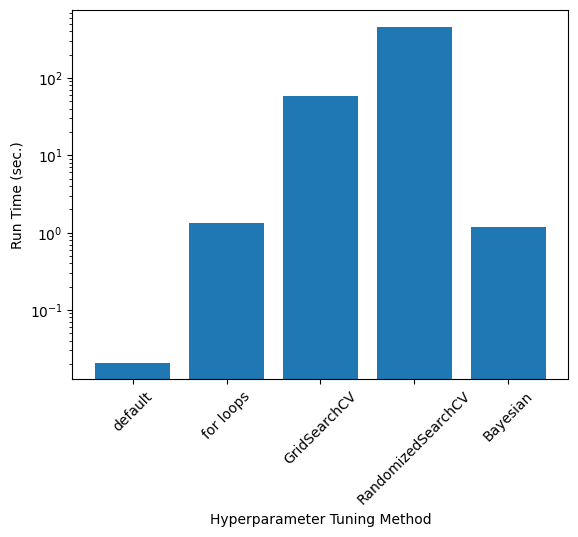
Part 2: Feature Engineering#
Feature engineering is the process of eliminating or altering given inputs in order to improve the model’s predictions
Design matrix/alter the inputs
Remove features that are not useful
Scaling the features or targets
import pandas as pd
import seaborn as sns
housing = fetch_california_housing()
housing_data = pd.DataFrame(housing.data, columns=housing.feature_names)
housing_data['target'] = housing.target
housing_data = housing_data.sample(1000)
Pairplots can give us initial ideas about the data set and and obvious relations
sns.pairplot(housing_data)
/Users/juliehartley/Library/Python/3.9/lib/python/site-packages/seaborn/axisgrid.py:118: UserWarning: The figure layout has changed to tight
self._figure.tight_layout(*args, **kwargs)
<seaborn.axisgrid.PairGrid at 0x164247e20>
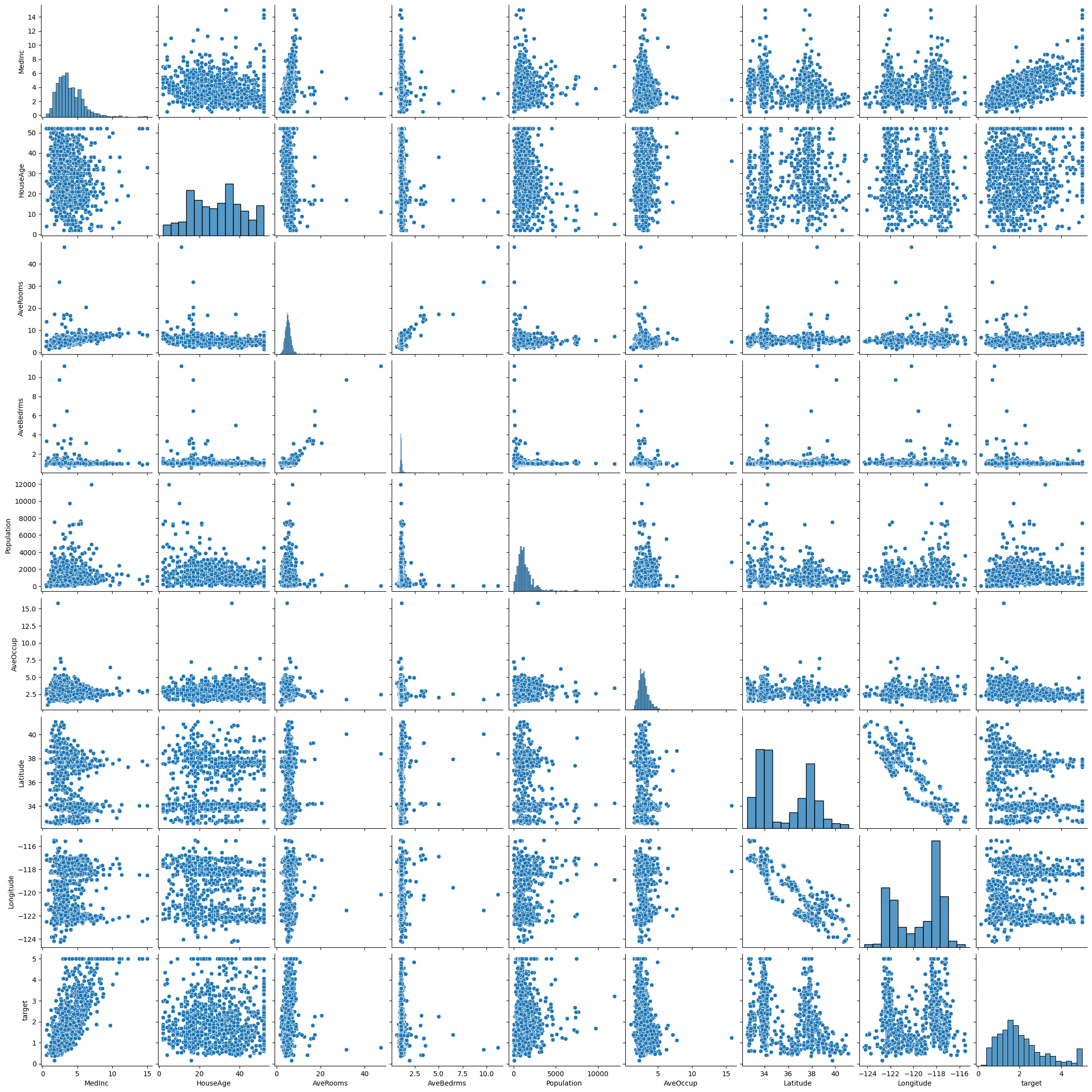
# Remove outliers from the data set and make the pairplot again
from scipy import stats
housing_data = housing_data[(np.abs(stats.zscore(housing_data)) < 3).all(axis=1)]
sns.pairplot(housing_data)
/Users/juliehartley/Library/Python/3.9/lib/python/site-packages/seaborn/axisgrid.py:118: UserWarning: The figure layout has changed to tight
self._figure.tight_layout(*args, **kwargs)
<seaborn.axisgrid.PairGrid at 0x1689a4070>
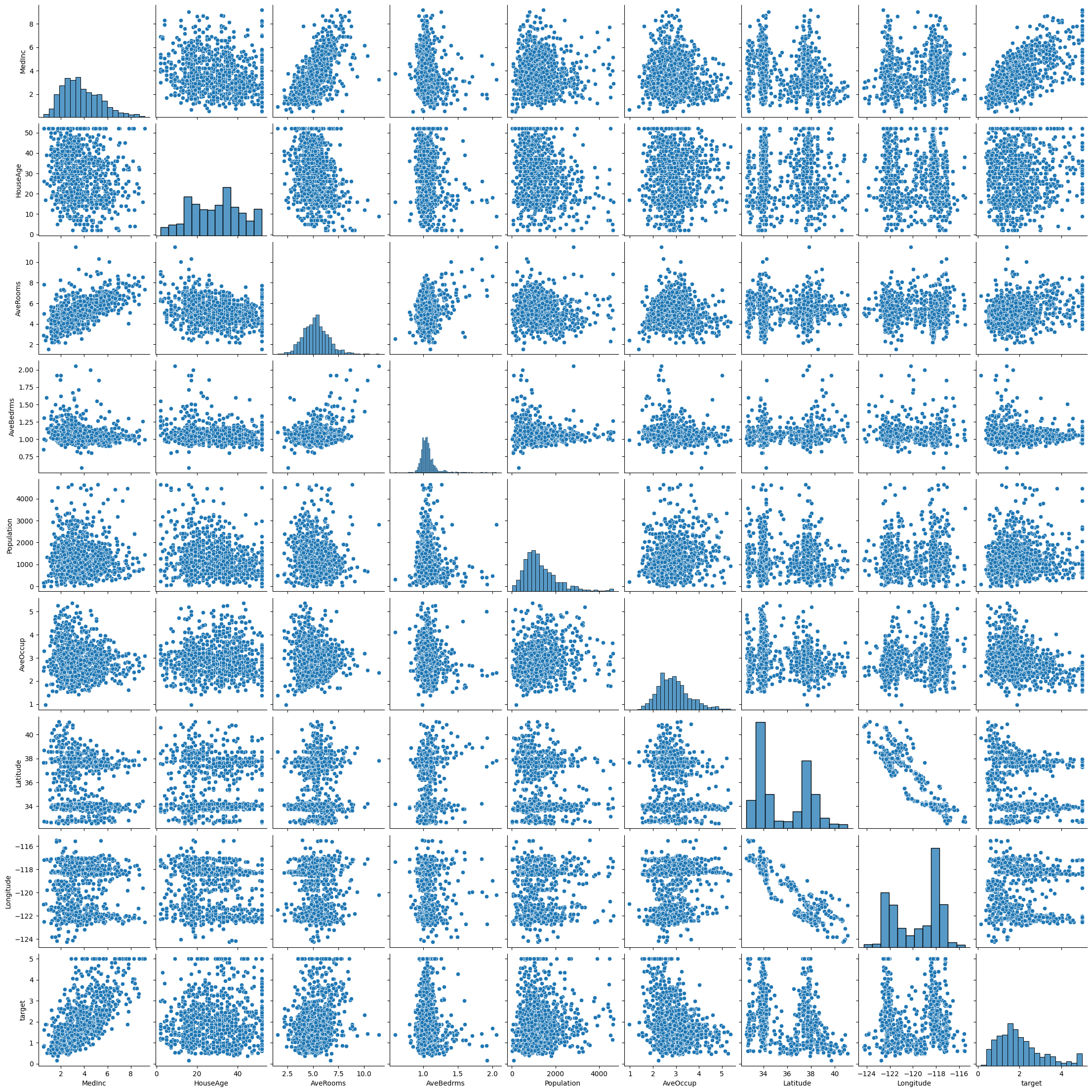
Correlation Matrix#
Correlation score = \(\sqrt{R2\ Score}\)
Values close to \(\pm1\) means that the two feautures are linearly related
correlation_matrix = housing_data.corr()
correlation_matrix
MedInc | HouseAge | AveRooms | AveBedrms | Population | AveOccup | Latitude | Longitude | target | |
---|---|---|---|---|---|---|---|---|---|
MedInc | 1.000000 | -0.176376 | 0.624278 | -0.182821 | -0.006504 | -0.118286 | -0.145364 | 0.051990 | 0.671977 |
HouseAge | -0.176376 | 1.000000 | -0.273970 | -0.171407 | -0.250648 | -0.000109 | 0.007118 | -0.100684 | 0.077059 |
AveRooms | 0.624278 | -0.273970 | 1.000000 | 0.292713 | -0.108061 | -0.049828 | 0.077900 | -0.022613 | 0.254047 |
AveBedrms | -0.182821 | -0.171407 | 0.292713 | 1.000000 | -0.080388 | -0.102396 | 0.132675 | -0.047917 | -0.121542 |
Population | -0.006504 | -0.250648 | -0.108061 | -0.080388 | 1.000000 | 0.170063 | -0.097118 | 0.070431 | 0.019527 |
AveOccup | -0.118286 | -0.000109 | -0.049828 | -0.102396 | 0.170063 | 1.000000 | -0.198600 | 0.214724 | -0.324090 |
Latitude | -0.145364 | 0.007118 | 0.077900 | 0.132675 | -0.097118 | -0.198600 | 1.000000 | -0.931286 | -0.198935 |
Longitude | 0.051990 | -0.100684 | -0.022613 | -0.047917 | 0.070431 | 0.214724 | -0.931286 | 1.000000 | 0.006876 |
target | 0.671977 | 0.077059 | 0.254047 | -0.121542 | 0.019527 | -0.324090 | -0.198935 | 0.006876 | 1.000000 |
plt.matshow(correlation_matrix)
plt.colorbar()
<matplotlib.colorbar.Colorbar at 0x28b885fd0>
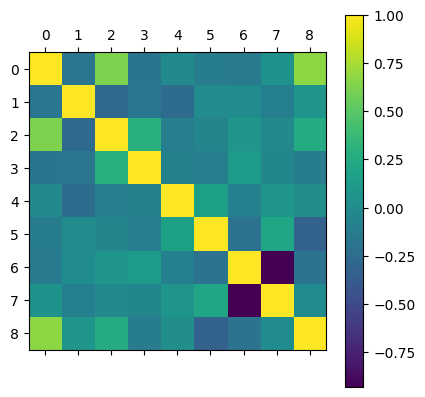
LASSO Regression for Feature Selection#
lasso = Lasso()
lasso.fit(X,y)
ypred = lasso.predict(X)
print(MSE(ypred,y))
lasso.coef_
0.638471842835069
array([ 0.06161184, -0. , 0. , -0. , 0.00011673,
-0. , 0. , 0. ])
Accuracy with All Data (Scaled)#
X = housing_data.drop(columns=['target'])
y = housing_data['target']
scaler = StandardScaler()
scaler.fit(X)
Z = scaler.transform(X)
X_train, X_test, y_train, y_test = train_test_split(Z,y,test_size=0.2)
bayesian_ridge = BayesianRidge()
bayesian_ridge.fit(X_train, y_train)
y_pred = bayesian_ridge.predict(X_test)
print('MSE:', MSE(y_pred, y_test))
MSE: 0.44933334607255193
Part 3: Nonlinear Models#
Kernel Ridge Regression (KRR)#
All previous models we have studied have been linear–capable of modeling linear patterns
Design matrices can only add some much functions
Many data sets will have a nonlinear pattern and thus we need a nonlinear model
Kernel ridge regression (KRR)
Support vector machines (SVMs)
Neural Networks
Kernel Functions#
Scikit-Learn kernels are found here
Linear: \(k(x,y) = x^Ty\)
Polynomial: k(x,y) = \((\gamma x^Ty+c_0)^d\)
Sigmoid: \(k(x,y) = tanh(\gamma x^Ty+c_0)\)
Radial Basis Function (RBF): \(k(x,y) = exp(-\gamma||x-y||_2)\)
Inputs to KRR algorithm are modified by the kernel function, thus giving the method nonlinearuty \(\longrightarrow\) kernel methods/trick allows linear methods to solve nonlinear problems
Kernel ridge regression is just ridge regression with the inputs modified by the kernel function
KRR Equations#
Form of predictions: \(\hat{y}(x) = \sum_{i=1}^m \theta_ik(x_i,x)\)
Loss function:\(J(\theta) = MSE(y,\hat{y}) + \frac{\alpha}{2}\sum_{i=1}^n\theta_i^2\)
Optimized parameters: \(\theta = (\textbf{K}-\alpha\textbf{I})^{-1}y\)
\(\textbf{K} = k(x_i, x_j)\)
Hyperparameter Tuning with Many Hyperparameters#
KRR has the same hyperparameter as ridge regression: \(\alpha\)
Each kernel function has 0-3 hyperparameters
The choice of kernel function is a hyperparameter
Hyperparameter tuning becomes more important as the number of hyperparameters in the model increases
Housing Data with Kernel Ridge Regression#
from sklearn.kernel_ridge import KernelRidge
X,y = fetch_california_housing(return_X_y=True)
X = X[:500]
y = y[:500]
scaler = StandardScaler()
scaler.fit(X)
Z = scaler.transform(X)
X_train, X_test, y_train, y_test = train_test_split(Z,y,test_size=0.2)
krr = KernelRidge()
krr.fit(X_train, y_train)
y_pred = krr.predict(X_test)
print("MSE:", MSE(y_pred, y_test))
MSE: 4.105741823609707
distributions = {'alpha':uniform(loc=0, scale=4), 'kernel':['linear', \
'polynomial', 'rbf', \
'sigmoid', 'laplacian'], \
'gamma':uniform(loc=0, scale=4),\
'degree':np.arange(0,10), 'coef0':uniform(loc=0, scale=4)}
krr = KernelRidge()
random_search = RandomizedSearchCV(krr, distributions,\
scoring='neg_mean_squared_error', n_iter=50)
random_search.fit(X_train, y_train)
print(random_search)
print(random_search.best_params_, random_search.best_score_)
---------------------------------------------------------------------------
KeyboardInterrupt Traceback (most recent call last)
Cell In [32], line 11
7 krr = KernelRidge()
9 random_search = RandomizedSearchCV(krr, distributions,\
10 scoring='neg_mean_squared_error', n_iter=50)
---> 11 random_search.fit(X_train, y_train)
12 print(random_search)
13 print(random_search.best_params_, random_search.best_score_)
File ~/Library/Python/3.9/lib/python/site-packages/sklearn/model_selection/_search.py:875, in BaseSearchCV.fit(self, X, y, groups, **fit_params)
869 results = self._format_results(
870 all_candidate_params, n_splits, all_out, all_more_results
871 )
873 return results
--> 875 self._run_search(evaluate_candidates)
877 # multimetric is determined here because in the case of a callable
878 # self.scoring the return type is only known after calling
879 first_test_score = all_out[0]["test_scores"]
File ~/Library/Python/3.9/lib/python/site-packages/sklearn/model_selection/_search.py:1753, in RandomizedSearchCV._run_search(self, evaluate_candidates)
1751 def _run_search(self, evaluate_candidates):
1752 """Search n_iter candidates from param_distributions"""
-> 1753 evaluate_candidates(
1754 ParameterSampler(
1755 self.param_distributions, self.n_iter, random_state=self.random_state
1756 )
1757 )
File ~/Library/Python/3.9/lib/python/site-packages/sklearn/model_selection/_search.py:822, in BaseSearchCV.fit.<locals>.evaluate_candidates(candidate_params, cv, more_results)
814 if self.verbose > 0:
815 print(
816 "Fitting {0} folds for each of {1} candidates,"
817 " totalling {2} fits".format(
818 n_splits, n_candidates, n_candidates * n_splits
819 )
820 )
--> 822 out = parallel(
823 delayed(_fit_and_score)(
824 clone(base_estimator),
825 X,
826 y,
827 train=train,
828 test=test,
829 parameters=parameters,
830 split_progress=(split_idx, n_splits),
831 candidate_progress=(cand_idx, n_candidates),
832 **fit_and_score_kwargs,
833 )
834 for (cand_idx, parameters), (split_idx, (train, test)) in product(
835 enumerate(candidate_params), enumerate(cv.split(X, y, groups))
836 )
837 )
839 if len(out) < 1:
840 raise ValueError(
841 "No fits were performed. "
842 "Was the CV iterator empty? "
843 "Were there no candidates?"
844 )
File ~/Library/Python/3.9/lib/python/site-packages/joblib/parallel.py:1088, in Parallel.__call__(self, iterable)
1085 if self.dispatch_one_batch(iterator):
1086 self._iterating = self._original_iterator is not None
-> 1088 while self.dispatch_one_batch(iterator):
1089 pass
1091 if pre_dispatch == "all" or n_jobs == 1:
1092 # The iterable was consumed all at once by the above for loop.
1093 # No need to wait for async callbacks to trigger to
1094 # consumption.
File ~/Library/Python/3.9/lib/python/site-packages/joblib/parallel.py:901, in Parallel.dispatch_one_batch(self, iterator)
899 return False
900 else:
--> 901 self._dispatch(tasks)
902 return True
File ~/Library/Python/3.9/lib/python/site-packages/joblib/parallel.py:819, in Parallel._dispatch(self, batch)
817 with self._lock:
818 job_idx = len(self._jobs)
--> 819 job = self._backend.apply_async(batch, callback=cb)
820 # A job can complete so quickly than its callback is
821 # called before we get here, causing self._jobs to
822 # grow. To ensure correct results ordering, .insert is
823 # used (rather than .append) in the following line
824 self._jobs.insert(job_idx, job)
File ~/Library/Python/3.9/lib/python/site-packages/joblib/_parallel_backends.py:208, in SequentialBackend.apply_async(self, func, callback)
206 def apply_async(self, func, callback=None):
207 """Schedule a func to be run"""
--> 208 result = ImmediateResult(func)
209 if callback:
210 callback(result)
File ~/Library/Python/3.9/lib/python/site-packages/joblib/_parallel_backends.py:597, in ImmediateResult.__init__(self, batch)
594 def __init__(self, batch):
595 # Don't delay the application, to avoid keeping the input
596 # arguments in memory
--> 597 self.results = batch()
File ~/Library/Python/3.9/lib/python/site-packages/joblib/parallel.py:288, in BatchedCalls.__call__(self)
284 def __call__(self):
285 # Set the default nested backend to self._backend but do not set the
286 # change the default number of processes to -1
287 with parallel_backend(self._backend, n_jobs=self._n_jobs):
--> 288 return [func(*args, **kwargs)
289 for func, args, kwargs in self.items]
File ~/Library/Python/3.9/lib/python/site-packages/joblib/parallel.py:288, in <listcomp>(.0)
284 def __call__(self):
285 # Set the default nested backend to self._backend but do not set the
286 # change the default number of processes to -1
287 with parallel_backend(self._backend, n_jobs=self._n_jobs):
--> 288 return [func(*args, **kwargs)
289 for func, args, kwargs in self.items]
File ~/Library/Python/3.9/lib/python/site-packages/sklearn/utils/fixes.py:117, in _FuncWrapper.__call__(self, *args, **kwargs)
115 def __call__(self, *args, **kwargs):
116 with config_context(**self.config):
--> 117 return self.function(*args, **kwargs)
File ~/Library/Python/3.9/lib/python/site-packages/sklearn/model_selection/_validation.py:686, in _fit_and_score(estimator, X, y, scorer, train, test, verbose, parameters, fit_params, return_train_score, return_parameters, return_n_test_samples, return_times, return_estimator, split_progress, candidate_progress, error_score)
684 estimator.fit(X_train, **fit_params)
685 else:
--> 686 estimator.fit(X_train, y_train, **fit_params)
688 except Exception:
689 # Note fit time as time until error
690 fit_time = time.time() - start_time
File ~/Library/Python/3.9/lib/python/site-packages/sklearn/kernel_ridge.py:195, in KernelRidge.fit(self, X, y, sample_weight)
192 ravel = True
194 copy = self.kernel == "precomputed"
--> 195 self.dual_coef_ = _solve_cholesky_kernel(K, y, alpha, sample_weight, copy)
196 if ravel:
197 self.dual_coef_ = self.dual_coef_.ravel()
File ~/Library/Python/3.9/lib/python/site-packages/sklearn/linear_model/_ridge.py:249, in _solve_cholesky_kernel(K, y, alpha, sample_weight, copy)
243 K.flat[:: n_samples + 1] += alpha[0]
245 try:
246 # Note: we must use overwrite_a=False in order to be able to
247 # use the fall-back solution below in case a LinAlgError
248 # is raised
--> 249 dual_coef = linalg.solve(K, y, assume_a="pos", overwrite_a=False)
250 except np.linalg.LinAlgError:
251 warnings.warn(
252 "Singular matrix in solving dual problem. Using "
253 "least-squares solution instead."
254 )
File ~/Library/Python/3.9/lib/python/site-packages/scipy/linalg/_basic.py:251, in solve(a, b, lower, overwrite_a, overwrite_b, check_finite, assume_a, transposed)
247 # Positive definite case 'posv'
248 else:
249 pocon, posv = get_lapack_funcs(('pocon', 'posv'),
250 (a1, b1))
--> 251 lu, x, info = posv(a1, b1, lower=lower,
252 overwrite_a=overwrite_a,
253 overwrite_b=overwrite_b)
254 _solve_check(n, info)
255 rcond, info = pocon(lu, anorm)
KeyboardInterrupt:
X,y = fetch_california_housing(return_X_y=True)
scaler = StandardScaler()
scaler.fit(X)
Z = scaler.transform(X)
X_train, X_test, y_train, y_test = train_test_split(Z,y,test_size=0.2)
# Using the best parameters from through one run of the
# RandomizedSearchCV method above
krr = KernelRidge(alpha= 0.35753693232459094, coef0= 3.2725118241858264, degree= 9, gamma= 0.14696609545731532, kernel= 'laplacian')
krr.fit(X_train, y_train)
y_pred = krr.predict(X_test)
print('MSE:', MSE(y_pred, y_test))
MSE: 0.23499324697787755
bayesian_ridge = BayesianRidge()
bayesian_ridge.fit(X_train, y_train)
y_pred = bayesian_ridge.predict(X_test)
print('MSE:', MSE(y_pred, y_test))
MSE: 0.5242651488478602