Creating Neural Networks with Tensorflow#
CSC/DSC 340 Week 8 Slides
Author: Dr. Julie Butler
Date Created: October 6, 2023
Last Modified: October 6, 2023
Keras and Tensorflow#
Keras and Tensorflow use to be seperate libraries but Keras is now apart of Tensorflow
In recent versions of Tensorflow, it has become hard to create and use neural networks without also using Keras
However, there are a few features that are still useful
Keras Neural Network With New Data Set#
MNIST Data Set
Input: images of handwritten digits (28x28 pixels)
Output: digit shown in the image
We will go into more detail on image manipulation and classification when we cover neural networks, but we can do a preliminary analysis on the MNIST data set with regular neural networks.
import matplotlib.pyplot as plt
from tensorflow.keras.datasets import mnist
## NOTE: The same data set is avaliable from Scikit-Learn
(train_images, train_labels), (test_images, test_labels) = mnist.load_data()
print(len(train_images))
60000
# Display a few sample images
num_samples = 5 # Change this to display a different number of images
plt.figure(figsize=(12, 4))
for i in range(num_samples):
plt.subplot(1, num_samples, i + 1)
plt.imshow(train_images[i], cmap='gray')
plt.title(f"Label: {train_labels[i]}")
plt.show()
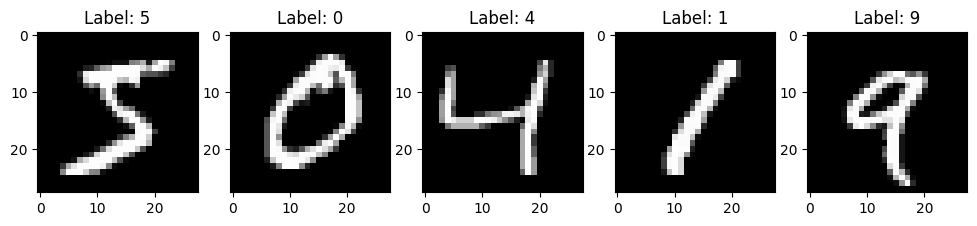
import numpy as np
import tensorflow as tf
from tensorflow.keras.datasets import mnist
from tensorflow.keras.models import Sequential
from tensorflow.keras.layers import Dense
from tensorflow.keras.utils import to_categorical
# Normalize pixel values to be between 0 and 1
train_images, test_images = train_images / 255.0, test_images / 255.0
# One-hot encode the labels
train_labels = to_categorical(train_labels)
test_labels = to_categorical(test_labels)
np.shape(train_images[i])
(28, 28)
def mnist_classification_model ():
# Define the model
model = Sequential()
# Add layers to the model
model.add(Flatten(input_shape=(28, 28))) # Flatten the 28x28 input images to a 1D vector
model.add(Dense(128, activation='relu')) # Fully connected layer with ReLU activation
model.add(Dense(64, activation='relu')) # Another fully connected layer with ReLU activation
model.add(Dense(10, activation='softmax')) # Output layer with 10 neurons for 10 classes (digits 0-9)
# Compile the model
model.compile(optimizer='adam', loss='categorical_crossentropy', metrics=['accuracy'])
return model
mnist_model = mnist_classification_model ()
---------------------------------------------------------------------------
NameError Traceback (most recent call last)
Cell In [10], line 1
----> 1 mnist_model = mnist_classification_model ()
Cell In [9], line 6, in mnist_classification_model()
3 model = Sequential()
5 # Add layers to the model
----> 6 model.add(Flatten(input_shape=(28, 28))) # Flatten the 28x28 input images to a 1D vector
7 model.add(Dense(128, activation='relu')) # Fully connected layer with ReLU activation
8 model.add(Dense(64, activation='relu')) # Another fully connected layer with ReLU activation
NameError: name 'Flatten' is not defined
history = mnist_model.fit(train_images, train_labels, epochs=10, batch_size=32, validation_split=0.2, verbose=1)
Epoch 1/10
1500/1500 [==============================] - 1s 835us/step - loss: 0.0065 - accuracy: 0.9980 - val_loss: 0.1808 - val_accuracy: 0.9755
Epoch 2/10
1500/1500 [==============================] - 1s 828us/step - loss: 0.0098 - accuracy: 0.9969 - val_loss: 0.1679 - val_accuracy: 0.9751
Epoch 3/10
1500/1500 [==============================] - 1s 810us/step - loss: 0.0079 - accuracy: 0.9975 - val_loss: 0.1697 - val_accuracy: 0.9745
Epoch 4/10
1500/1500 [==============================] - 1s 808us/step - loss: 0.0059 - accuracy: 0.9982 - val_loss: 0.1896 - val_accuracy: 0.9735
Epoch 5/10
1500/1500 [==============================] - 1s 805us/step - loss: 0.0112 - accuracy: 0.9965 - val_loss: 0.1665 - val_accuracy: 0.9784
Epoch 6/10
1500/1500 [==============================] - 1s 808us/step - loss: 0.0052 - accuracy: 0.9983 - val_loss: 0.1901 - val_accuracy: 0.9747
Epoch 7/10
1500/1500 [==============================] - 1s 874us/step - loss: 0.0094 - accuracy: 0.9973 - val_loss: 0.1842 - val_accuracy: 0.9757
Epoch 8/10
1500/1500 [==============================] - 1s 857us/step - loss: 0.0063 - accuracy: 0.9982 - val_loss: 0.2077 - val_accuracy: 0.9749
Epoch 9/10
1500/1500 [==============================] - 1s 809us/step - loss: 0.0072 - accuracy: 0.9976 - val_loss: 0.1842 - val_accuracy: 0.9770
Epoch 10/10
1500/1500 [==============================] - 1s 838us/step - loss: 0.0053 - accuracy: 0.9984 - val_loss: 0.1975 - val_accuracy: 0.9758
# Evaluate the model on the test data
test_loss, test_accuracy = model.evaluate(test_images, test_labels)
print(f"Test accuracy: {test_accuracy * 100:.2f}%")
313/313 [==============================] - 0s 464us/step - loss: 0.0953 - accuracy: 0.9721
Test accuracy: 97.21%
# summarize history for accuracy
plt.plot(history.history['accuracy'])
plt.plot(history.history['val_accuracy'])
plt.title('model accuracy')
plt.ylabel('accuracy')
plt.xlabel('epoch')
plt.legend(['train', 'validation'], loc='upper left')
plt.show()
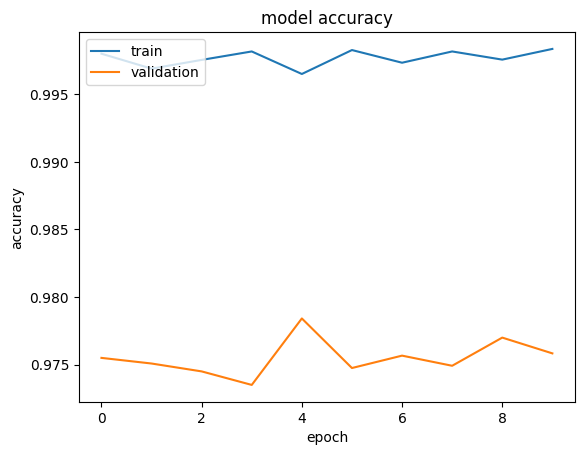
Custom Loss Functions#
Tensorflow Variables#
GPU Support#
Preprocessing Layers#
Flatten Layer#
Normalization Layer#
CategoryEncoding Layer#
Image Preprocessing Layers#
Saving Trained Models#
Other Tensorflow Customizations#
Tensorflow offers many other customizations besides the ones discussed here:
Custom Activation Functions
Custom Initialization Schemes
Custom Layers
Custom Training Loops
These are unlikely to be used in this course, but are good to know about for the future